The Victorian Institute of Forensic Medicine (VIFM) – which also operates as Monash University’s Department of Forensic Medicine, performs autopsy services for all deaths reported to the Victorian State Coroner.
It holds a globally unique data collection – more than 75,000 full-body computed tomography (CT) scans of dead people, an archive that increases by 7000 cases a year. All causes of death are represented, including traumatic injury, homicide and suicide. All age groups and genders are represented.
The database also holds more than two million digital photographic images, including external and internal injuries, pathological conditions, and external whole-body images.
It’s a huge bank of potentially game-changing information; however, the ability to search it all to find new information is limited.
Unlocking potential
When Monash’s Professor Chris Bain, from the Faculty of IT, first heard about it and pondered the possibilities of it in machine-learning or artificial intelligence, he was gobsmacked.
“My jaw hit the floor,” he says. “When I picked it up, I realised the potential.”
Professor Bain is the University’s first Professor of Practice in Digital Health. He’d only been in the job about two months – this was two years ago – when he listened to a presentation by the deputy director of VIFM, Associate Professor Richard Bassed, who described the database.
“VIFM have a huge database of scans and photographs,” Professor Bain says. “This is great knowledge, so we’re trying to work with them over time to build them a library. This is very valuable for them, but the bigger picture is they become the final source of truth – the gold standard, if you like – on the question of, ‘How did someone die?’.
“The vision,” he says, “ is to advance the science of forensic medicine using much more computational power and far fewer archaic, human methods.”
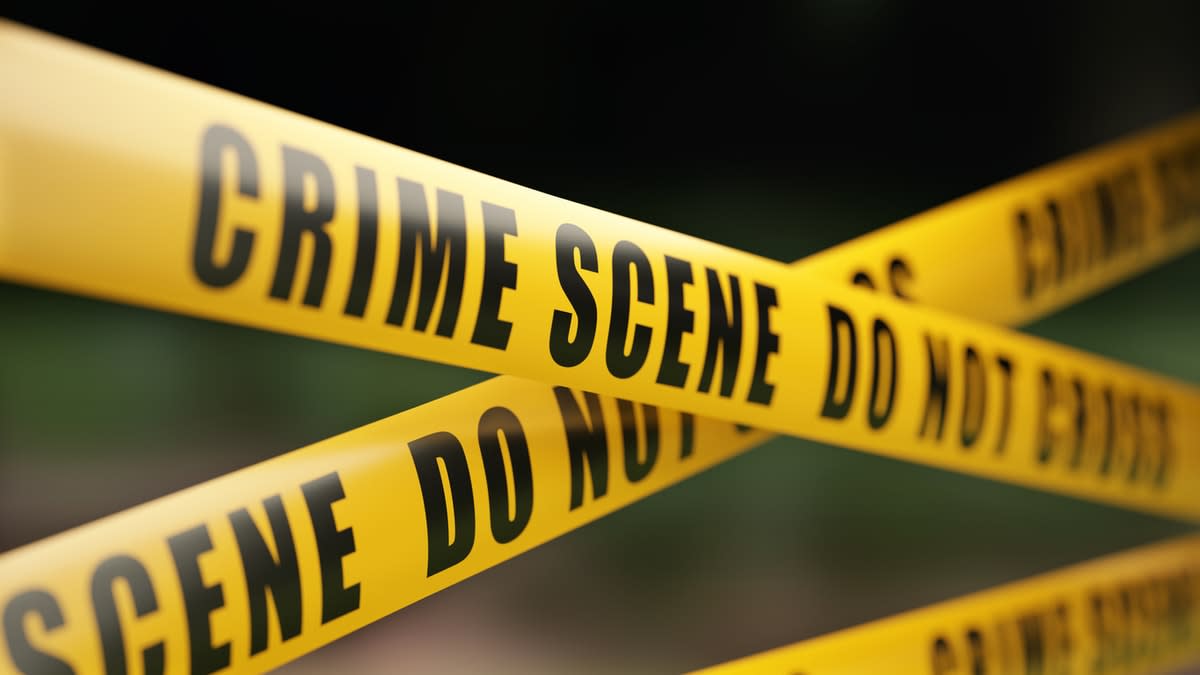
There are four projects underway in the collaboration. An IT and biomedical research company, Leidos, has contributed $150,000 to the first project (part of a wider partnership the Monash Institute of Medical Engineering) on bullet trajectories – that is, what bullets do and where they go inside bodies. Leidos want products that have a military health application.
The project – which is staffed and began work six months ago – aims to use machine learning to create a digital 3D model of human anatomy, including bullet entry and exit wounds. The scans and photographs at VIFM are the raw material, and eventually the idea is to have algorithms to map trajectories of bullets, find bullet fragments, and determine the calibre and range from which the gun was fired.
“We want machine learning to learn repeating patterns about what happens with certain bullet injuries,” Professor Bain says. “For example, what do high-velocity bullets do to the chest? This could be valuable for military frontline care. It’s limited funding at this stage, but that doesn’t stop us exploring it in an early research phase.”
“The vision is to advance the science of forensic medicine using much more computational power and far fewer archaic, human methods.”
Associate Professor Bassed said ballistics in forensic medicine involved “fairly basic analytic techniques” that hadn’t changed for 100 years.
“Before we had CT imaging, we were using x-rays to produce a 2D view of someone’s body, which made localising projectiles and fragments difficult without conducting an internal examination. Trajectory was determined using basic techniques such as long probes to determine a projectile’s path. Current imaging techniques can’t differentiate between bullet fragments and foreign metal objects, such as a pacemaker or dental fillings.
“This technology will allow us to make a 3D digital reconstruction of a shooting victim that we can then slice in multiple planes and directions using advanced computer graphics, including the use of augmented reality. We can then apply machine learning to determine trajectory and projectile fragmentation, and create a 3D-printed model that can potentially be used as evidence in a court of law.”
Legal advantages
Professor Bain says the project signals a step in a potential move away from physical autopsies. He says the legal angle – that machine learning may make autopsies more accurate – is compelling.
“One of the criticisms of forensic evidence as it’s presented now is that it’s too dependent on the opinion of an expert. You could argue that every time you have a human interpretation you’re open to legal attack.”
He says that in the future there may be less need for post-mortems on shooting victims, as the technology would scan the body rather than the body being physically opened up and examined.
Associate Professor Bassed said the Department of Forensic Medicine had a number of grant applications pending that relate to forensic medical case analysis and death or injury prevention using machine learning as a tool for analysis and prediction.